AlphaFold reiterates link between AI and biology
In recent weeks, DeepMind’s solution to the grand “protein folding problem” of biology has enthralled scientists in biology and computer science across the world. AlphaFold, the AI system tasked with this challenge, can accurately determine protein structure (and therefore function) with significantly less time and equipment than existing methods. It’s not an overstatement to say that this represents a paradigm shift in many fields of scientific discovery.
Among other areas of significance, AlphaFold’s ability to rapidly elucidate protein structure could shave countless years and billions of dollars off the drug discovery process. Imagine a world where a pandemic-causing pathogen has multiple viable therapeutic targets within weeks of discovery thanks to the ability to determine the structure of disease-causing proteins in days.
Beyond AlphaFold
But, AlphaFold is only one of many recent examples in applying the computational magic of AI to biology.
A team at Google recently developed an augmented-reality microscope (ARM) that overlays information like predicted tumor regions for metastasis detection onto live views from the lens, allowing those who use ARMs to leverage, in just seconds, the expertise of the many pathologists who helped train AI modules to power these tools. Helping scientists detect cancer in real-time speeds up pathology work and may ultimately help doctors save more lives, particularly in regions of the world with less on-site pathology expertise. We at Biodock are particularly excited about this increasing interplay between AI and human scientific expertise and what it means for the future of medicine.
Leveraging AI doesn’t make human knowledge obsolete, but rather it helps biologists glean more information from their data with greater convenience. Scientists at UCSF trained a neural network to take unlabeled cell images and extrapolate locations of sub-cellular structures (ex. dendrites in neuronal cells), potentially removing the need for tedious procedures like immunofluorescent staining . This method could help researchers gain, “almost for free,” additional context about cell samples under study.
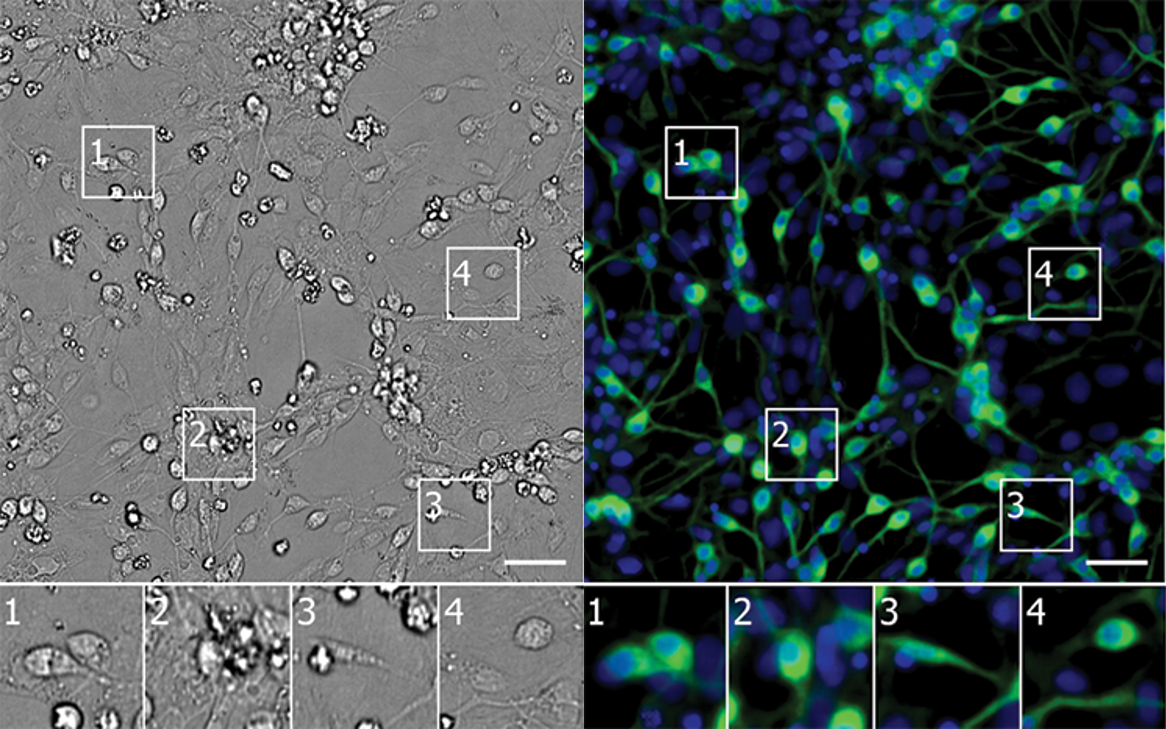
Beyond image analysis, Verily Life Sciences, Alphabet’s life science subsidiary, created a deep learning tool called DeepVariant that can identify single nucleotide polymorphisms (SNPs) - variations in the nucleotide sequence within the genome - with significantly greater accuracy than existing methods. DeepVariant is one of many AI-powered tools that will help biology realize the power of fast, accurate genome sequencing to help physicians improve clinical outcomes (ex. predicting how effective therapeutics will be based on genomic indicators).
Expanding the promise of AI in biology in the 2020s
One of the most interesting promises of artificial intelligence has always been to automate away time-consuming and tedious tasks. Time is your most precious resource as a human, and AI can give us more of it. Here are a few examples of this automation paradigm in science and beyond:
- Manufacturing quality control - Manufacturing is a massive, multi-trillion dollar industry in the US, and a large part of the manufacturing process involves extensive quality control. Much of this can now be automated thanks to recent advances in machine learning. For example, BMW employs computer vision tools in its factories to help compare vehicle order data with live images of newly produced cars to automate the tedious process of cross-checking order accuracy. With these tools, biopharmaceutical companies can also benefit from reduced waste and more accurate and consistent production lines.
- Processing clinical data - Even to this day, clinical studies often involve patients recording relevant information in paper logs, which are tedious to comb through for large-scale studies. Leveraging computer vision and other AI tools to parse through and interpret handwritten data would significantly increase the efficiency of such tasks for the doctors and scientists involved.
- Writing emails - Few people enjoy writing emails, yet it sucks away an unseemly amount of time for workers, including doctors and scientists. People staggeringly spend more than five hours a day on email. Thanks to tools like OpenAI’s new GPT-3 language generator, we may not have to anymore. The team at Otherside AI is building a sophisticated email generation tool to give us back this time.
We believe biological data analysis is next. For the uninitiated, analyzing biological data (imaging, sequencing, etc.) is often far more tedious than some expect. The move-fast, spontaneous-insight-driven portrayal of scientific discovery in Hollywood is juxtaposed with the unglamorous, but entirely necessary, reality of biological research: hours of pipetting, days of manual image annotations, and weeks of repeat experiments.
Biodock’s mission to help realize this vision
“Deep-learning tools are evolving rapidly, and labs will need dedicated computational expertise, collaborations or both to take advantage of them.”
- Sarah Webb, PhD in Nature
Here at Biodock, our mission is to build the future of biological data analysis by collaborating with labs to automate the extraction of information from image data using cloud AI. Tasks as disparate as segmenting cells, calculating epidermal nerve fiber density, and analyzing organoids are integral to many tasks in biology but often require time-consuming manual analysis. We hope to return valuable time scientists spend on these tasks, so they can work on realizing the many promises of biological science, from developing next-generation cancer therapeutics to engineering pandemic-resolving vaccines.
_____
For more information about Biodock’s product suite, reach out to nicholasb@biodock.ai.