While examining molded petri dishes after a vacation in 1928, Sir Alexander Fleming noticed that the periphery of the mold had no bacterial growth. He would come to find that the mold was secreting an antibacterial agent later called penicillin. This drug discovery revolutionized medicine. Penicillin is one of multiple drugs that were discovered by accident. Today, however, the serendipity Fleming encountered is seldom experienced. Drug discovery has proven extraordinarily difficult. The potential landscape of small molecules for drugs numbers in the tens of millions, making finding effective treatments like finding a needle in a haystack. Moreover, 90% of drugs that are even successful in reaching clinical trials fail (Figure 1). To account for this bottleneck, drugs are first screened for efficacy on cells. Significant effort has been invested in increasing the throughput of assessing drugs for therapeutic efficacy. One burgeoning means of doing this is through image-based profiling, where relevant markers are used to visualize cellular features that report drug effects which are analyzed in a high-throughput fashion through computer vision. With advancements in deep learning applications in image analysis, image-based profiling is becoming an increasingly appealing and viable option for assessing drug efficacy and is poised to accelerate the process of drug discovery.
Here, I will discuss how image-based profiling slots into contemporary methods for drug discovery and how it is already being used to identify drug effects and mechanisms.
Table of Contents
1. The basics of drug discovery
2. Drug screening vs profiling
3. Image-based profiling methods
4. Image-based profiling in practice
5. Outlooks for the future of image-based profiling in drug discovery
Drug discovery begins with identifying molecules with favorable molecular mechanisms of action which have reproducible and robust effects
As I alluded to previously, the vast landscape of potential molecules for drugs makes initial testing on mammals and humans impractical and unethical. Therefore, cultured cells make for more suitable subjects to test the initial effects of drugs on. They can be acquired and grown for a relatively low cost and their high numbers make statistical analyses on drug effects viable. It also increases the throughput of potential drugs to be tested. Furthermore, the cellular resolution affords the dissection of the mechanism of action of the drug. This is critical to establish as it will inform its therapeutic potential and shed light on potential concerns such as toxicity.
Once candidate drugs show effects in vitro, animal testing can be initiated in species of increasing similarity to humans such as rodents and non-human primates. Clinical trials can commence if the drug shows favorable therapeutic outcomes in animal models of the disease. This includes several stages that evaluate the safety and efficacy of the drug in increasing cohorts of humans over time (Figure 1).
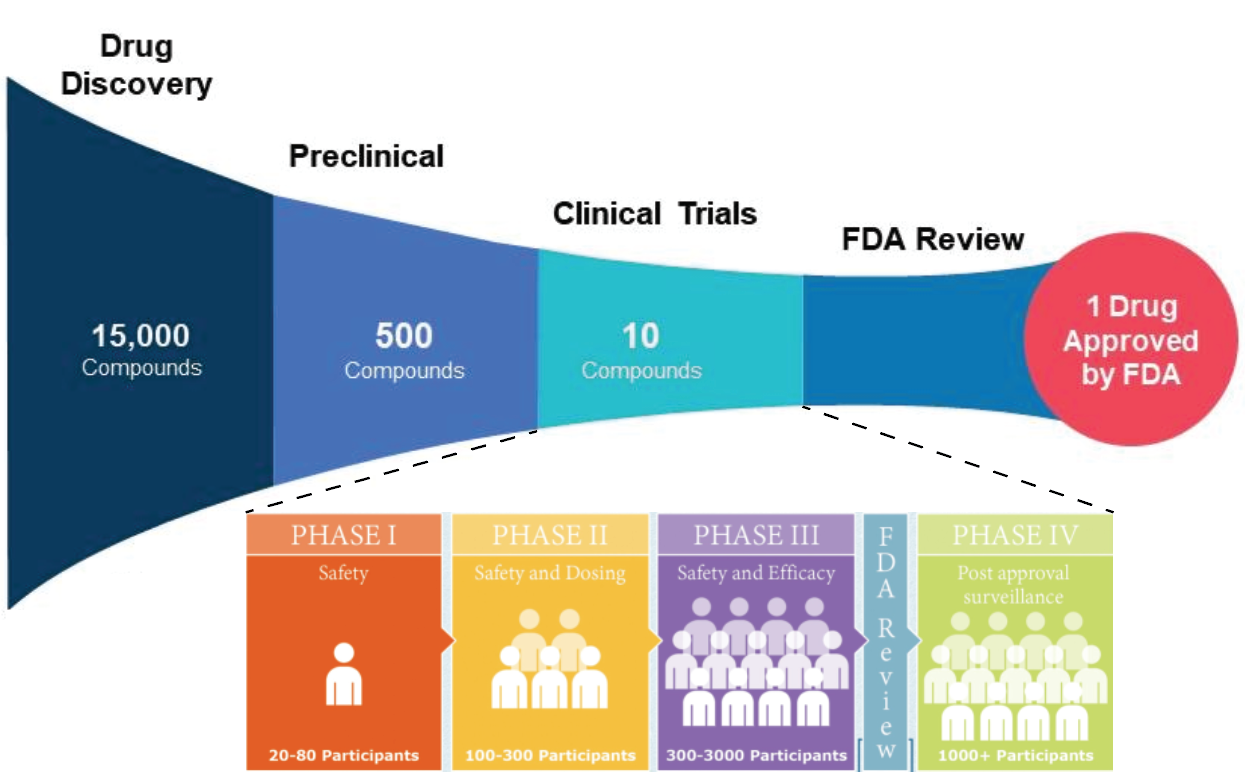
Although many (including myself earlier) admonish the high failure rate of clinical trials, a drug passing through preclinical trials constitutes a vastly more imposing bottleneck. One of the thousands of candidate drugs might make it, representing a fraction of a fraction of a percent. This bottleneck begs the urgency for the development of more efficient high-throughput approaches. To determine the preliminary efficacy and molecular mechanism of candidate drugs, there are two high-throughput approaches: Screening and profiling.
Screening vs. Profiling: Going to the grocery store with a list or an empty stomach
Do you go to the grocery store with a curated list? Or do you go, assess what is in the store, and pick out what your stomach tells you to? These discrete approaches mirror that of the distinction between screening and profiling in drug discovery.
Screening is a commonly used approach. In drug screening, a readout for the disease that is both easily analyzable and closely approximates the disease-associated response is used. This readout can be a variety of things. In target-based screening, this readout can be the interaction with a specified disease-related protein. One example of this is screening for molecules that interact with amyloid beta which is a protein enriched in the neurodegenerative disease Alzheimer’s. This readout can also represent a molecular event. In pathway-based screening, a process along a biochemical pathway that is associated with the disease can be assayed. For example, in Tay-Sachs disease, a mutation in an enzyme involved in the metabolism of glucose leads to the build-up of a cytosolic-toxic biproduct. A pathway screen for Tay-Sachs might entail screening for drugs that influence the activity along the same metabolic pathway. Although screening is an efficient and targeted tool for drug discovery, it necessitates the presence of a clear and easily measurable molecular marker for the disease. This might not always be feasible, nor might we always have identified and validated features of the disease state. This motivates an alternative approach in drug discovery called profiling.
Profiling complements the weakness of screening in that one can capture a multiplicity of cellular and molecular features which offers a more comprehensive perspective from which to assess the disease state and drug efficacy. In profiling, a sample such as cells can be represented by their profile which constitutes a variety of potentially relevant features which can be obtained through commonly used staining techniques. Profiling benefits from the use of staining since one can label multiple cellular features and simultaneously examine these separate streams of information to gather a multidimensional perspective on cell state which could be influenced by disease and or drug. One such commonly used stain is Cell Painting which is a sequence of six stains that allow morphological profiling of discrete cellular features such as mitochondria and nuclei (Figure 2). This is an example of a phenotypic screen, where directly observable changes to morphology report disease state and or drug effect. Armed with cell painting, one has a rich set of morphological features from which to interrogate drug efficacy and could enumerate previously unvalidated drug effects lost to simpler target and pathway screens. Performing a phenotypic screen using stains to generate cellular profiles from images has become widely used and is referred to as image-based profiling.
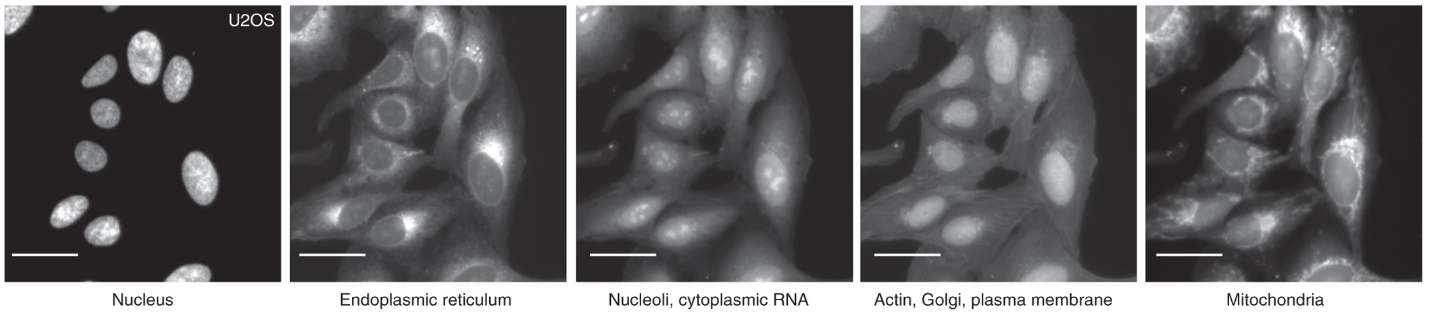
Machine learning integration into image-based profiling represents a viable and competent approach for drug discovery
Image-based profiling is growing in popularity and automated means of analyzing high-content images have grown tangentially. To increase the throughput of image-based profiling and make effective use of the high-dimensional profiles generated, pharmacologists have partnered with computer scientists to develop machine learning-based approaches that discover and identify drug effects. I mentioned before that Cell Painting affords a high-dimensional image set from which to perform a phenotypic screen or profile cells. The phenotypes and combinations thereof to analyze are vast. Not to mention that subtle differences might be inaccessible with normal manual quantification methods. Since manual annotation and or bulk analyses are incompatible with the magnitude of potential drugs to test, automated methods to analyze this data are paramount. What tasks might these models perform in theory?
Cell painting stains the genetic and structural components of the cell as well as important organelles such as mitochondrion. Models can be trained to generate ROIs for readily identifiable boundaries such as the cell membrane and the nucleus. These ROI masks can then be applied to other channels and extract information about other cellular compartments (Figure 3). For example, extracting geometric quantities from the model predicted cell outline could inform how a drug influences cellular structure which could be further confirmed by looking at the distribution of intensity for cytoskeletal proteins such as actin in another channel. Depending on the disease, this might shed light on a putative mechanism of action for the drug such as influencing molecules involved in actin polymerization. A sequential target screen for this drug could thusly commence. Although this example illustrates how a model performing cellular outlining in image-based profiling of cell painted samples could sequentially lead to a putative mechanism of action for a drug, it is merely theoretical. This begs the question: how is image-based profiling being used in practice?
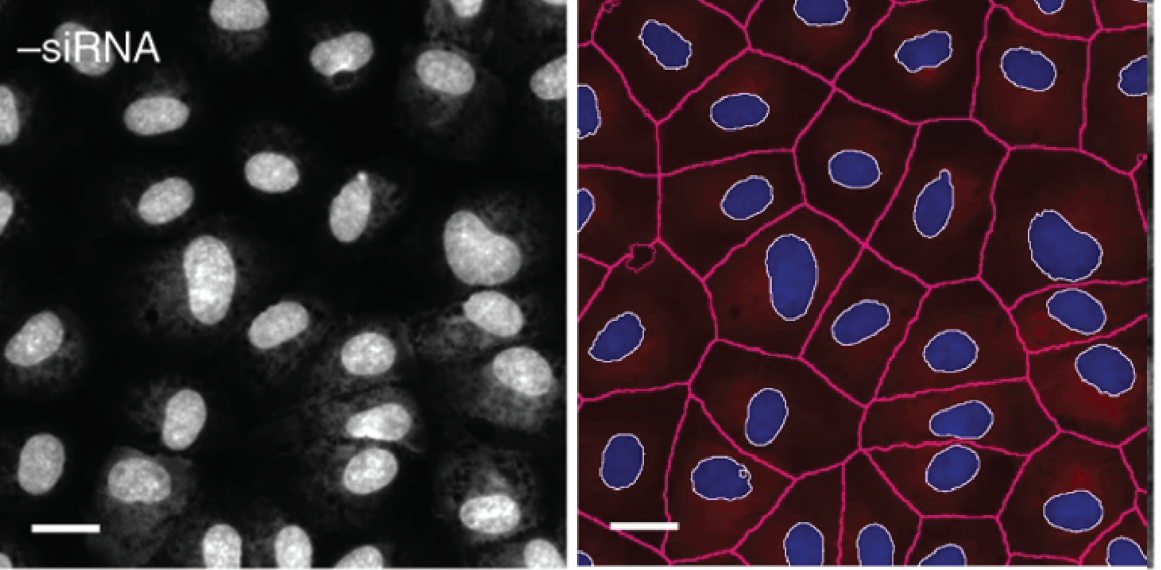
Image-based profiling using machine learning can accurately predict the pharmacological activity of compounds
Understanding the pharmacological activity of compounds is imperative for developing a mechanism of action and explaining its potential therapeutic effects. Can we infer the activity of compounds from biological images of cell samples? Image-based profiling offers an appealing outlet to do this since the high content of images and multiple stains can predict activity across multiple intracellular targets. Simply put, a picture is worth a thousand target screens. One team put this to the test. Simm et. al 2018 aimed to predict the activity of compounds from images. To do this, they extracted morphometric features from cell images using unsupervised segmentation methods. With activity data from a glucocorticoid receptor HTI assay, they trained a convolutional neural network to predict the activity of the compound (Figure 4). Not only did they find that they could accurately predict activity, but they demonstrated a 5-fold increase in compound hit rate using image-based profiling compared to typical target screening methods.
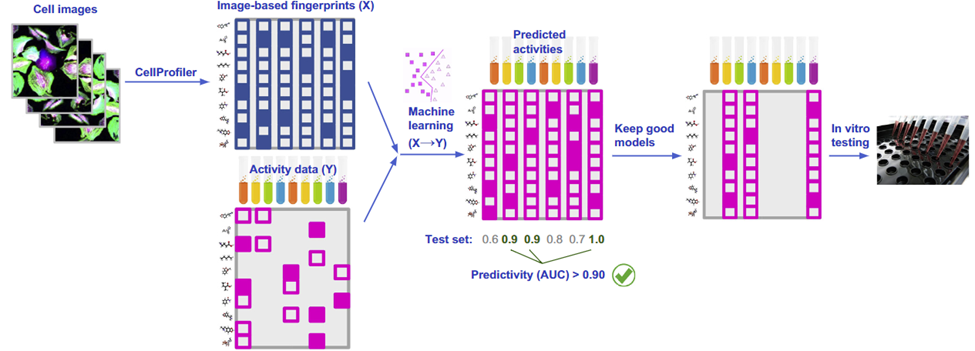
Data sets to assay activity using image-based profiling are becoming available. For example, one group created ‘The Cell Image Library’ repository which accumulates data from 919,874 five-channel fields of view representing 30,616 tested compounds.
In addition to the activity of a compound, evaluating its toxicity is an essential step in the drug discovery process. How might image-based profiling also be used to evaluate the toxicity of potential drugs?
Image-based profiling using machine learning can accurately predict the toxicity of compounds
Just because a compound achieves a desired effect on the disease state does not mean it is safe for human use. In fact, 17% of clinical trials in phase three fail due to safety concerns. Thus, evaluating the toxicity of a compound is equally as important as determining its efficacy. The broad capture of image-based profiling makes it highly sensitive to detecting cellular effects and thus predicting the toxicity of compounds.
One group utilized image-based profiling to determine the nephrotoxicity of xenobiotics on kidney cells. They tested 44 reference compounds on cultured human renal proximal tubular cells (PTCs). They then quantified 129 image features pertaining to cellular, nuclear, and cytoarchitectural elements using automated pipelines based on random forest algorithms for single- and multi-feature detection. They achieved over 80% accuracy in predicting nephrotoxicity. Moreover, image-based profiling using the cell-painting technique has been used to efficiently perform large-scale screening on environmental chemicals to assess toxicity.
Image-based profiling using machine learning can infer the mechanism of action of a compound
Establishing the mechanism of action (MOA) of a drug is vital for proceeding with a clinical trial and is required by the FDA. Insights into the molecular targets of a drug can inform its therapeutic potential and potential off-target effects. Typically, non-image-based assays have been used to assess MOA such as testing for phosphorylation. Recently, however, image-based profiling has become an accurate tool with which to infer MOA. By imaging putative molecular and cellular targets, altered image features can be quantified and extracted. This strategy is already being used in oncological research.
For example, images of cells in a model for colorectal carcinoma subjected to cell-cycle modulator drugs were analyzed using a custom classifier. They were able to infer the kinase activity of compounds. Another group developed a novel image-based profiling assay to measure aspects of cancer such as mitochondrial apoptosis, DNA damage, and cell cycle disruption. Beyond oncology, image-based profiling has been used for general target- and pathway-screening to determine MOA in nearly three thousand compounds. Using image-based readouts such as shape, intensity, and texture, one group was able to generate fingerprints which served as the basis for their clustering algorithm. They were able to disambiguate discrete clusters associated with individual intracellular targets such as tubulin and intracellular signaling pathways such as mTOR. Although the compounds they used had an established MOA, the author's clustering methods were able to extract novel compound target associations, emphasizing the power of image-based profiling in unbiasedly determining MOA.
The future of computer-based automation in drug discovery has optimistic room for improvement
With the increasing statistical and analytical power of deep learning models and clustering algorithms, image-based profiling possesses an optimistic future. Although cell painting offers a high-content base from which to extract image features, most studies to date have used simple image features such as cellular and nuclear morphology. Although this testifies to the potential of image-based profiling, improved segmentation and quantification of subcellular compartments and organelles can even further improve the predictive power and throughput of image-based profiling in drug discovery. This might depend on developing novel imaging assays and or using higher-resolution imaging methods. Overall, however, image-based profiling has reshaped how we conceptualize the method of drug discovery and is becoming widely available and adopted.