Single-cell transcriptomics has grown tremendously in popularity and accessibility within the life sciences since the first proof-of-principle paper was published in 2009. Chosen as the Nature Methods method of the year in 2013, commercial entities (Illumina, Pac Bio, 10x Genomics) now largely dominate the single-cell library preparation and sequencing ecosystem. Spatial sequencing, the younger sibling of scRNAseq (and 2021 method of the year!), one-ups scRNAseq in allowing researchers to map transcripts onto cellular and tissue architecture and understand the context of mRNA expression. Although fully spatially resolved single-cell transcriptomes are not yet possible, many researchers in this fast-moving field are optimistic that it’s coming soon. Similarly, computational methods that leverage spatial information—taking into account cellular neighbors, for instance—are still in early days, but new methods are reported at a rapid pace. Although managing data across different platforms and finding optimal methods to integrate spatial and non-spatial data remains a challenge, many groups have applied a combination of scRNAseq and spatial sequencing to report new insights around things like brain architecture and embryo development. In a recent paper by Ma et. al. from the Modlin group at UCLA, researchers report new insights into how the immune system controls leprosy within granulomas using this mix of spatial and non-spatial sequencing and dataset integration.
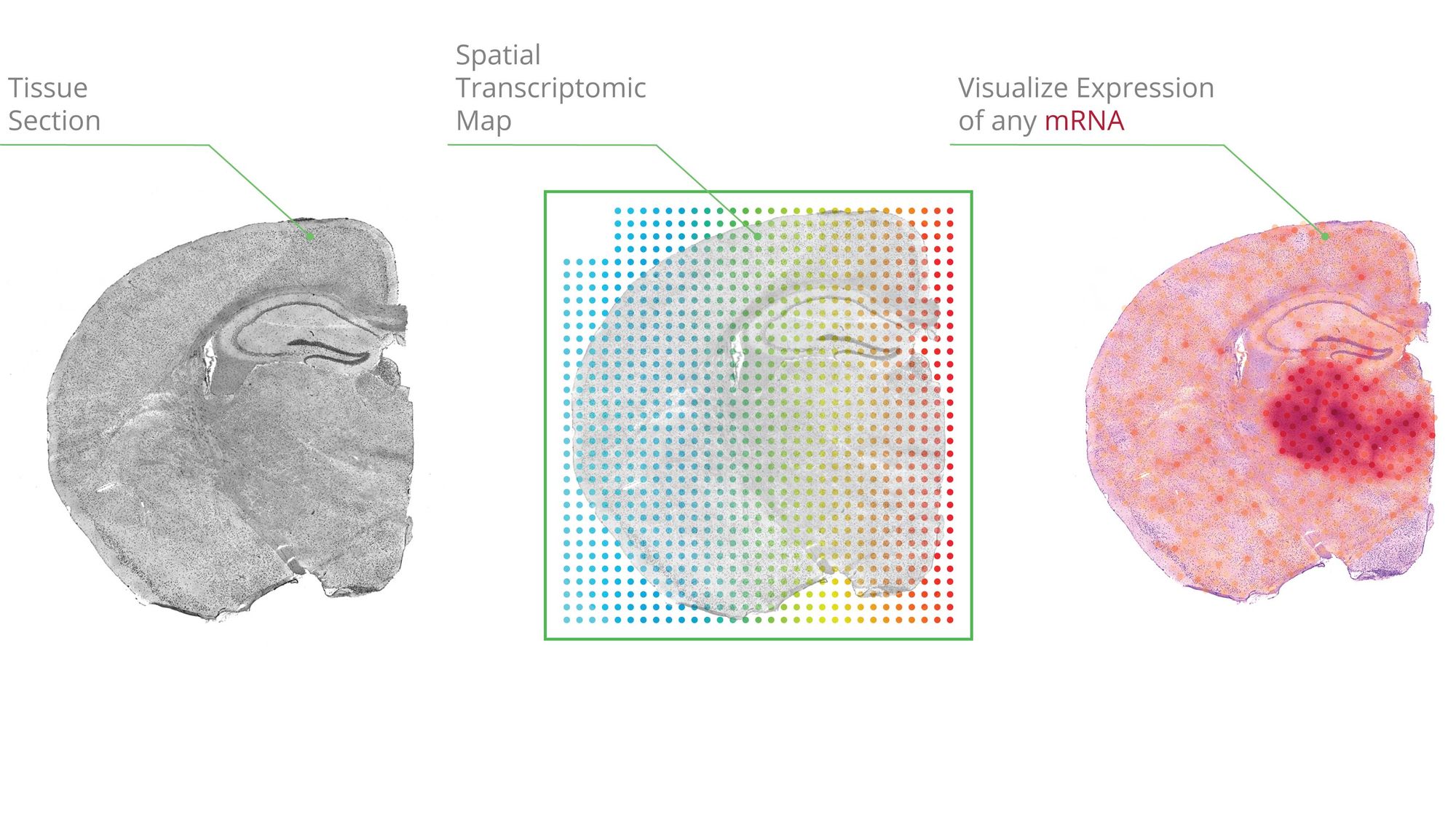
Granulomas—immune-cell-rich structures that form within chronically infected tissues—are the body’s attempt to sequester and kill pathogens that have evaded an acute immune response. Although most commonly associated with tuberculosis, granulomas are also a hallmark of leprosy, caused by the bacterium Mycobacterium leprae. The human response to M. leprae spans a dynamic continuum ranging from a self-limiting infection referred to as tuberculoid (T-lep) to chronic infection (lepromatous leprosy, or L-lep) characterized by abundant skin lesions. Patients can “upgrade” from L-lep to T-lep spontaneously and begin to mount an effective antimicrobial defense to clear infection, a process referred to as a reversal reaction (RR). Ma et al. investigate the cellular architecture and functional characteristics of granulomas associated with RR in order to further our understanding of what makes a granuloma effective.
The authors first perform scRNAseq on skin biopsy samples from five patients with L-lep and five patients that had undergone reversal to T-lep (RR samples). Clustering analysis identified 12 general cell types shared by all patients. These included myeloid cells and T-lymphocytes, which generally make up the majority of a granuloma, as well as skin-specific cells such as fibroblasts and keratinocytes. The authors then subset the myeloid and T-cell compartments to look more closely at immune cell phenotypes. Uncontrolled infection L-lep samples contained significantly more macrophage subsets expressing antiviral response genes (annotated as ML1 cells) as well as macrophages expressing altered lipid metabolism genes (ML2 cells). On the other hand, samples from patients undergoing reversal were abundant in dendritic cells and M1-like macrophages (ML4 cells). An additional macrophage subset, ML3, represented a transition population between the L-lep dominant ML2 subset and the RR dominant ML4 subset. The authors then used pseudotime analysis to infer a trajectory from the ML2 macrophage state to the ML4 state, with ML3 as a transition state. The cytokines IL1β and IFNγ were identified as upstream drivers of this trajectory, turning on dozens of antimicrobial genes with known direct bactericidal effects again M. leprae.
Next, the authors generated a complementary spatial sequencing dataset from skin biopsy samples containing granulomas using the Visium platform (10x Genomics). Using cell-specific expression matrices from the existing scRNAseq dataset, cell type composition for each spatially sequenced spot was determined. The concordance of cell type-specific antimicrobial gene expression between the single-cell and spatial datasets was high, and spatial sequencing allowed the authors to visualize the architecture of the various immune subsets and expression of key antimicrobial genes within the granuloma structure. Notably, in RR and T-lep granulomas, ML3 (transition) and ML4 (M1-like) macrophages formed the core of a relatively organized structure, with lymphocytes and dendritic cells in the “mantle” zone surrounding this core. In contrast, in L-lep granulomas, ML2 macrophages and antiviral macrophages predominated, perhaps limiting the extent of antimicrobial gene expression by immune cells in the vicinity and contributing to uncontrolled infection. Importantly, the combination of spatial and single-cell transcriptome sequencing identified new roles for fibroblasts and keratinocytes, found on the periphery of the granuloma structure. Antimicrobial gene expression in these subsets was spatially mapped onto cell-type-resolved granuloma maps. The authors suggest these non-immune cell types may play an important role in antimicrobial gene expression and bacterial control.
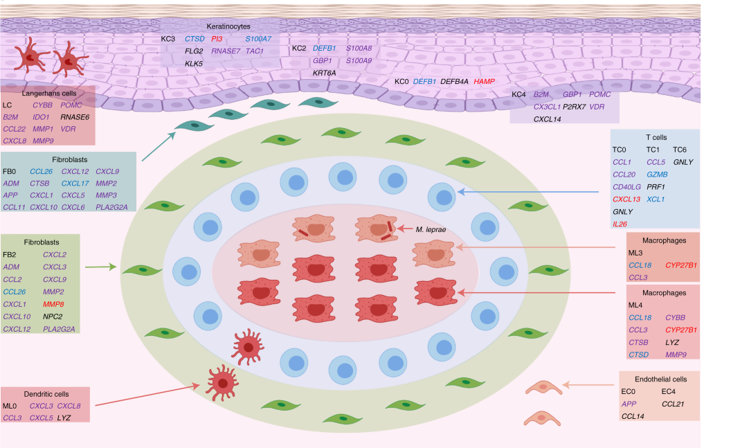
These exciting findings generate many new avenues of investigation. For example, do dendritic cells or T cells influence the antimicrobial expression of nearby fibroblasts and keratinocytes? Similarly, are changes in cell-cell interaction related to increased expression of IL1β and IFNγ? Additional technical progress in spatial sequencing, such as obtaining higher resolution to approach single-cell localization and increasing the number of genes detected per imaged region, will go a long way towards answering questions like this. Similarly, development of spatial-sequencing-specific analysis methods that leverage cell-proximity information will also serve to clarify the underlying biology of an evolving granuloma.