Heterogeneity is a characteristic all biologists are familiar with. In its negative manifestations, we struggle against variations in our experiments, our model systems, and our tools. On the other hand, some of us make a career of studying heterogeneity. This is particularly the case in the field of cancer research, where accumulating knowledge over the past few decades has driven home the massive variations in tumors within a given type of cancer, between different cancers, and between different patients. Cancer researchers have long understood the importance of personalized treatments and the importance of predicting response based on disease characteristics, but we are only just starting to achieve this level of patient care. A critical tool for interrogating malignancies and their local environments is bioimaging, which can visually reveal the relationships and interactions between tumor cells and non-malignant cells in the tumor microenvironment (TME), as well as chemotherapy or immunotherapy drug effects on cancerous lesions. The TME consists of immune cells, endothelial cells, and cancer-associated fibroblasts which influence tumor growth and behavior, albeit through little-understood mechanisms. Although the TME is known to be a critical factor in predicting malignant progression, we still don’t know which cell types are pro-cancer and which are protective, or the variables that predict drug responsiveness.
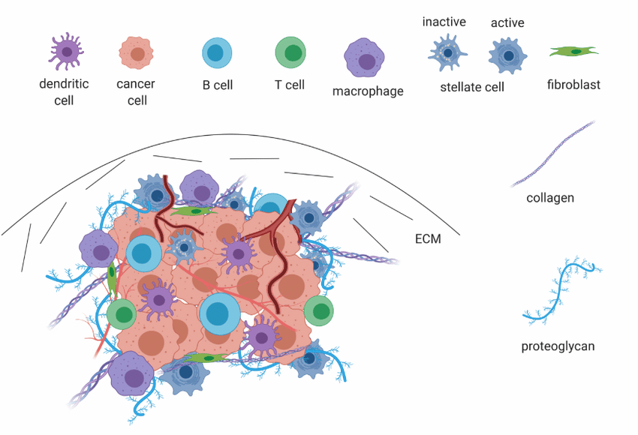
Grünwald et al. make major progress towards untangling cancer heterogeneity with a recent paper in Cell, “Spatially confined sub-tumor microenvironments in pancreatic cancer”. The authors leverage an ambitious array of -omics approaches, including proteomics, bulk RNAseq, and single-cell RNAseq, multiple imaging modalities such as high-parameter immunohistochemistry and histological analysis, and numerous in vitro tools such as patient-derived organoids to assemble a comprehensive investigation of TME heterogeneity in pancreatic ductal adenocarcinoma (PDAC).
PDAC is the most common type of malignancy that arises from the pancreas, and currently stands as the fourth most frequent cause of cancer-related mortality worldwide. This cancer is particularly aggressive, tends to be detected relatively late, and is highly heterogenic even when compared to other cancers. Although multiple groups have published on the PDAC TME and it’s thought that immunosuppressive cells in this compartment are major factors in immunotherapy responsiveness, clinicians don't have a good handle on which clinical or histological features should be used to inform treatment options. In this work, the authors aim to identify common phenotypes of the PDAC TME across patients and disease stages, and leverage their imaging, sequencing, and cellular data to assign functional relevance and discover predictive variables for cancer progression and treatment response.
The sample set investigated here consisted of 143 treatment-naïve histological resections as well as 210 pre-treatment biopsies from advanced cases of PDAC. As a first step, the authors identified 3 major TME phenotypes that accounted for the majority of TME heterogeneity through systematic review of histological images. These 3 grouping were defined as the following:
· “Deserted” regions with skinny fibroblasts, loose fibers, and keloid/myxoid features
· “Reactive” regions with plumper fibroblasts with enlarged nuclei, not many acellular components, and rich inflammatory infiltrate
· “Intermediate” regions somewhere in between these two extremes
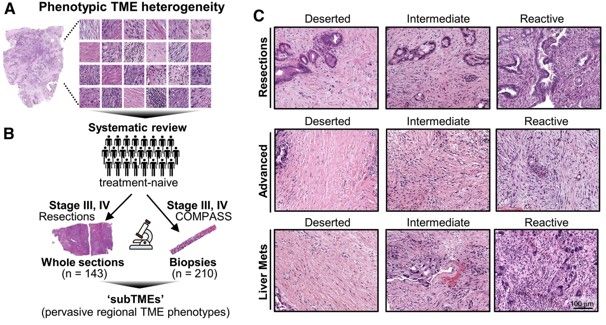
All of these phenotypes were found to be present to varying degrees in all stages of PDAC examined. Individual tumor biopsies/resections could be classified as one main TME, where >50% of the tumor area fell into one phenotype, and could also be assessed for heterogeneity by evaluating whether multiple TMEs were present. TMEs that occurred within the same tumor were named sub-tumor microenvironments (subTMEs). subTMEs were frequent: ~50% of resections and ~70% of biopsies contained multiple TMEs. Interestingly, patient survival was not found to correlate with main TME type, but rather degree of tumor heterogeneity. The more heterogenous the TME, the worse prognostic/survival outcome. This finding spurred the authors to dive deep into intratumoral heterogeneity and profile the composition and function of these identified subTMEs. To this end, laser capture microdissection was used to cut out specific subTMEs from 32 treatment-naïve tumor samples. subTME samples were split into two aliquots and sent for profiling by shotgun proteomics and bulk RNA-seq. Both profiling methods showed a significant degree of grouping by subTME category with the intermediate phenotype appearing to be a transitional population between the deserted and reactive states. The authors therefore collapsed the intermediate and reactive categories into a single grouping for rest of their data analysis. The RNA/protein pairs that were differentially expressed between the deserted and intermediate/reactive subTMEs were known factors involved in tumor/stromal interaction as well as cancer-associated fibroblast (CAF) subpopulation markers. GSEA analysis was performed to inform possible subTME-specific biological characteristics, and it was found that deserted TMEs were characterized by enrichment of extracellular matrix signaling and humoral immunity (i.e. antibody/B-cell mediated immunity), while reactive subTMEs were defined by fibroblast-activating/immunomodulatory factors, pro-inflammatory cytokines, and cellular immunity-related gene sets including innate immunity, antigen presentation, TCR signaling.
These results were in line with the histological features of these different TMEs, and the authors next chose to look at specific cellular composition via a deep-phenotyping platform. Using the sample set of 143 PDAC tumor resections, the researchers first mapped the subTME landscape of each sample and then applied a 25-marker immunohistochemistry panel followed by digital quantification to investigate subTME composition across patients and across patient-paired subTMEs. As expected based on sequencing and proteomic data, the reactive/intermediate subTME samples had increased staining for immune cells while the deserted subTME had high staining for collagen and a nonsignificant trend towards B cell enrichment.
In a parallel set of experiments the authors expanded on a preliminary finding from the RNAseq/proteomic dataset, where it appeared that distinct cancer-associated fibroblast subtypes segregated to different subTMEs. Cancer-associated fibroblasts from the two identified subTME groupings were isolated from 13 new PDAC specimens for single-cell characterization and functional assays. Single-cell analysis showed that deserted-type fibroblasts were enriched in growth-related genes, while reactive-type fibroblasts were enriched in immune-related genes. In line with these data, the growth patterns of fibroblast monolayers from reactive subTME fibroblasts were more motile, and deserted subTME fibroblasts grew faster. These data suggested that cancer-associated fibroblast subtypes may define the phenotype of the cellular communities that build up around them: inflammatory fibroblasts recruit immune cells and drive reactive subTME development, while highly proliferative fibroblasts contributed to deserted subTMEs with increased extracellular matrix content but few other infiltrating cells.
Finally, the authors address the big question: how do subTMEs affect tumor cell behavior and overall cancer progression/treatment response? RNAseq and proteomics analysis of cancerous cells revealed that reactive subTME-associated tumor cells were enriched for genes encoding cell cycle progression factors and oncogenic signaling, while deserted subTME-origin tumor cells were enriched for cellular metabolism and protein secretion genes. Correspondingly, reactive-dominant TME drove a more basal-like cancerous phenotype and shortened survival in this patient sample, while the deserted TME state appeared to support tumor differentiation and lead to longer survival. With respect to chemotherapy effectiveness, the authors found that conditioned media from deserted-type fibroblasts increased chemotherapy drug resistance in a majority of patient-derived organoids compared to media from reactive fibroblasts, indicating that deserted TMEs drive poorer chemotherapy response. Indeed, deserted TME-dominant patients had poorer response to first-line chemotherapy. The effect of neoadjuvant therapy on the TME landscape was similarly significant: a higher proportion of deserted TME-dominant tumor biopsies were observed post-chemo than in stage-matched treatment-naïve biopsies, indicating that extended treatment may drive TME evolution.
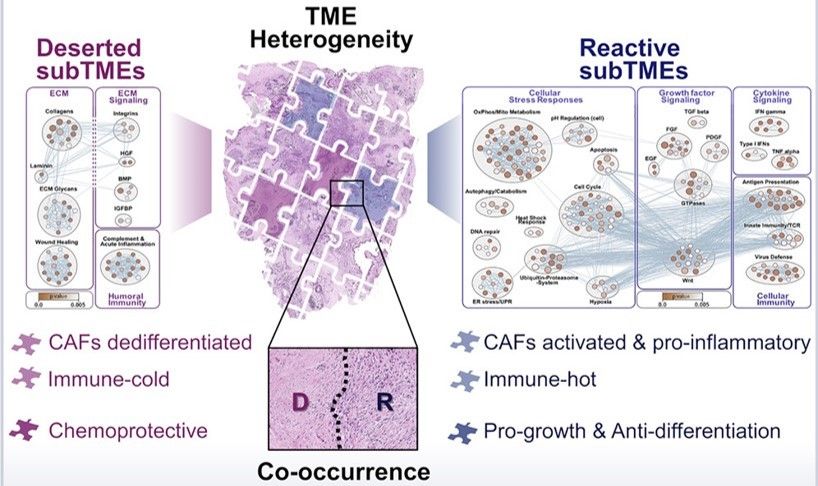
In sum, Grünwald et al. report some very exciting findings with major potential for patient benefit and clinical application. Most fundamentally, they establish that PDAC TMEs can be meaningfully described by two major phenotypic groupings: reactive and deserted. Reactive TMEs are inflamed and tumor-promoting but also more chemo-sensitive. Deserted TMEs correlate with tumor differentiation but predict poor treatment responses. Tumors that existed within a mix of both reactive and deserted TME therefore get the benefit of both chemoprotection and increased growth, leading to worse patient outcome than tumors with a single TME type. These are the kinds of projects that Biodock is excited to work on—our platform enables cell segmentation and marker analysis pipelines on a cloud-based system for unlimited storage space and immediate access. Have a large dataset of IHC images that need to be quantified? We can do it--reach out for more details!