We know about the AI algorithms moderating our social media experience or suggesting new shows on Netflix. If you’re a scientist, you might use deep learning in your own research or have seen it applied in new papers from the past few years. One group of researchers is bringing deep learning to that workhorse of bioimaging: confocal microscopy. Confocal imaging is heavily used and appropriate for many biological questions, but has some technical limitations that make it inappropriate for certain applications. If you need to image thick sections measured in the millimeters or if your work requires lengthy timeseries on the order of hours, your samples will likely undergo photobleaching and the images may exhibit degradation of resolution due to diffraction and signal scattering. In a recent paper from late 2021, Wu et al. present a new microscopy platform that builds on confocal and address the limitations listed above using deep learning approaches and technical innovations.
The authors first introduce a new laser setup, combining scanning in straight lines with multiview (i.e. multiple objective) imaging in order to reduce signal scattering and blurring. They arrange three objectives to serially scan the sample using specially engineered laser scanners. Reconstruction of the fluorescence signal from this triple-view setup increased resolution both laterally and axially as compared to traditional single-view confocal imaging, and was tested on fluorescent beads and labelled microtubules. The authors then applied this triple-view system on fixed cells that had been physically expanded via expansion microscopy sample preparation to visualize subcellular structures on the nanometer range. Again, the triple-view setup achieved better suppression of distortion than conventional confocal. Finally, a fixed intact C. elegans (nematode) larva with nuclear staining was imaged. The triple-view approach gave clearer views of subcellular structures with less scattering and background at depth, and it did it 26x faster than point-scanning confocal.
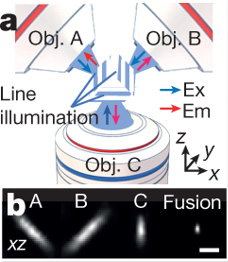
Interestingly, the authors used this larval imaging experiment as a vehicle to improve on their deconvolution algorithm and identify each individual stained nucleus in the worm body. Using a deep-learning routine combined with manual editing, they segmented all 2000-some nuclei, a task that is currently impossible with point-scanning confocal alone. The triple-view system can also be used on living cells: human carcinoma cells and contractile cardiomyocytes were successfully imaged in this work. The contractile motion of the cardiomyocytes caused motion blur and photobleaching, which prompted the authors to again apply deep learning to clean up the final images. Using a sequential neural network approach, a low signal-to-noise (caused by bleaching or blur) image was denoised by net 1, and the output of net 1 was fed into net 2, which deconvoluted net 1 output to predict a high signal-to-noise image. This two-step approach worked better than using a single neural net to both denoise and predict output. A third neural net was developed to segment and count C. elegans nuclei. The authors report that while traditional single-view confocal images captured less than half the true number of nuclei, the 2-step clean-up neural net approach applied to the same single-view images found nearly all of them.
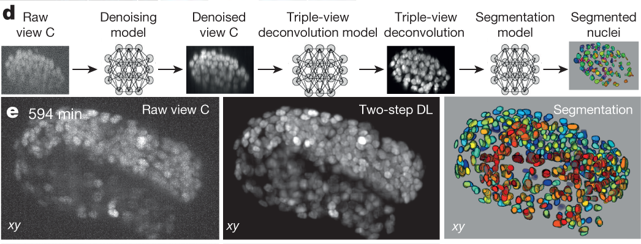
Finally, Wu and colleagues bring multiview imaging to structured illumination microscopy (SIM), a super-resolution technique (see here for more info on SIM). They develop a system in which the sample is scanned along 3 orthogonal directions, and 5 images per direction are collected and processed to create a 3D super high-resolution reconstruction. The major gain of this SIM improvement is the ability to image thick, densely labelled samples, because the multiple-objective setup is very good at blocking out-of-focus light. And of course, the authors applied deep learning to enhance resolution in this application as well.
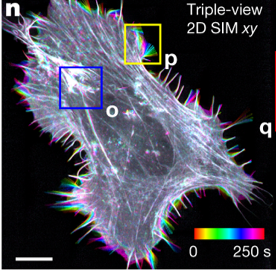
In this ambitious and important work, Wu and colleagues applied multiview imaging and deep learning to improve both confocal and super resolution microscopy, representing a novel use of AI to extend imaging duration, depth, and resolution. The authors note that their work could be extended by applying multiview imaging to other types of microscopy with line illumination, with cleared tissue sample preparation techniques (see here for more detail on this approach), or to a longer-wavelength scope to image even thicker samples. Have your own super-resolution (or otherwise) large imaging datasets? Interested in using deep learning to streamline your analysis or get more from your data? Take a look at Biodock's custom AI-driven pipelines here!