Take a walk down the probiotics aisle of your local pharmacy and you’ll find an overwhelming number of products claiming to change, improve, strengthen, or otherwise modify your gut microbiome. For a market whose value is somewhere north of $50 billion dollars, current clinical trials and meta-analyses in the field actually underscore how little we know about the complex interactions between microbes, both between resident microbial communities in the gut (or skin, oral cavities, etc), as well as between resident communities and ingested foreign bacteria. The first generation of microbiome studies involved large-scale metagenomic sequencing of fecal or gut biopsy samples, generating laundry lists of species and some information about their relative abundances. However, we now know that the bacteria that colonize us mostly don't exist as single isolated cells (termed “planktonic”) but rather form and live within a complex higher-order structures called biofilms.
Biofilms are aggregations of bacterial cells embedded in a polymer matrix of both host- and microbial-derived molecules. Biofilms in the human gut are adherent to the mucus layer that coats our GI tract, to the cellular epithelium, or to food particles. Biofilms can be polymicrobial—that is, contain multiple bacterial species—and possess both spatial and temporal organization. Although biofilms are a major feature of the healthy human gut, they can also form in undesirable locations such as on a surgical implant, within a lung infected with pathogenic bacteria, or on a urinary catheter. Biofilms are such a widespread mode of microbial organization because they confer many benefits to the cells living within them, including resistance to antibiotics, tolerance of environmental stressors such as desiccation, and protection from immune cells and other bactericidal host-produced components.
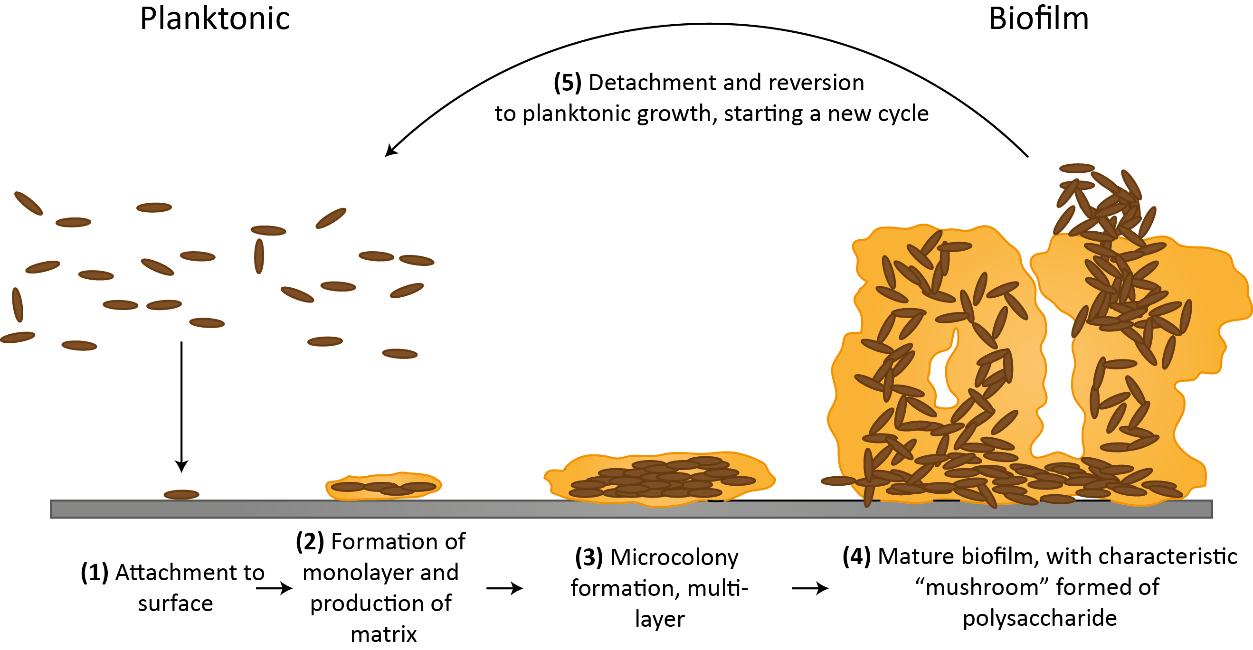
For those trying to characterize and model gut microbiome community interactions, lists of species and their abundances are not sufficient to build an accurate theoretical representation of this system. Because a biofilm has emergent properties not found in a population of planktonic bacteria and consists of global scale interactions within the community and between spatially-linked communities, mathematical models seeking to predict biofilm dynamics and perturbation responses would greatly benefit from further research in this field. Understanding what our mucosal and pathogenic biofilms look like—including which microbes are present, how they interact in space and time, how different treatments affect biofilm composition, and what gene programs biofilm-resident cells are turning on—has major translational potential and medical impact.
One suite of approaches for spatially mapping microbial communities might be familiar to researchers in other fields: CLASI-FISH, HiPR-FISH, MiPACT and others are RNA-FISH-based techniques to visualize bacterial cells. Although eukaryotic RNA-FISH approaches typically look at expression of various genes of interest (see this explainer for more technical detail), prokaryotic RNA-FISH is generally used to ID bacterial genera and species on the basis of 16S rRNA sequence. The 16S ribosomal sequence is hypervariable between microbial taxa but also highly conserved across domains, and therefore allows the researcher to design oligo probes specific to a certain bacterial genera or species as well as probes which capture all members of a larger taxonomic grouping. rRNA sequences are also present in relatively high copy number within bacterial cells, making them ideal for high FISH signal.
For imaging microbial communities with small numbers of unique species, off-the-shelf RNA-FISH based on 16S probes is good enough. However, this approach is limited to the number of optically distinct fluorophores that the microscope in use can detect (typically 4 for an epifluorescence scope). However, since many human-associated biofilms contain much more than 4 species, combinatorial labelling approaches have recently been developed to increase the number of species imaged at once. CLASI-FISH (short for combinatorial labelling and spectral imaging FISH), first described in 2011, is a technique in which each species is assigned a two-fluorophore barcode and imaged using laser scanning confocal microscopy. The fluorescence signals are then segmented and demultiplexed using linear unmixing and pre-determined reference spectra to determine the taxonomic label for each cell. Using 6 distinct fluorophores and a 2-color barcode, 15 different unique labels were generated and used to identify members of a human dental plaque sample as well as a 15-member human gut microbiome community established in a gnotobiotic mouse model. The authors then performed proximity analysis to extract statistically significant information about bacterial association frequencies. More recently, CLASI-FISH was used to identify 17 unique genera in human tongue samples and led to a hypothesized model for the growth, competition, and microniche-specific proliferation of oral biofilm communities.
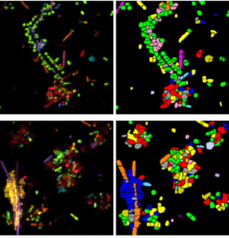
An obvious extension of CLASI-FISH for higher-complexity samples is to increase the number of fluorophores used to barcode a species. HiPR-FISH (high phylogenetic resolution FISH)—a new technique reported earlier this year—takes this approach, using combinations of 10 fluorophores to identify 1023 different E. coli isolates and a 7-fluorophore barcode to identify 65 genera in human oral biofilm samples. Given the much larger number of spectra to unmix, the analysis requirements of HiPR-FISH are substantially different than that of 2-bit barcode techniques. Each bacterial cell was assigned a spectral barcode by concatenating the emission spectra of the five excitation lasers used, and decoded using a machine learning classifier trained on individual E. coli isolates labelled with a single barcode. Furthermore, the authors developed a novel algorithm to distinguish background from signal for each HiPR-FISH image pixel in order to improve single-cell segmentation of these densely packed, diversely shaped microbial communities. HiPR-FISH mapping of mouse gut microbiome samples revealed significant disruption in the spatial organization of biofilms in response to antibiotic treatment, an example of the power of this technique.
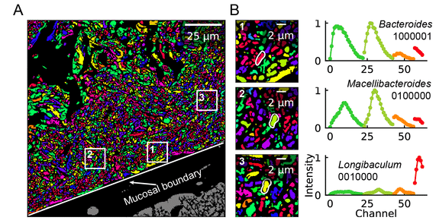
Given the pace of technology development for mapping the microbiome, it will be exciting to see the applications of these and related techniques in the near future. Colonic biopsy samples from patients with Crohn's disease, timecourse samples from individuals treated with oral antibiotic courses, geographically diverse gut samples from populations with different lifestyles and diets: there are countless questions to be answered! If you're a researcher working in this space, take a look at Biodock's custom storage, segmentation and analysis pipelines--it could just cut your data processing time significantly!